The Advent, Evolution, and Current state of “Data Translators”
Introduction
With Data being constantly glorified as the most valuable asset organizations can own, leaders and decision-makers are always looking for effective ways to put their data insights to use. Every time customers interact with digital products, millions of data points are generated and the opportunity loss of not harnessing these data points to make better products, optimize revenue generation, and improve customer footprint is simply too high to ignore. The role of “Data Translators” began to emerge in analytics and data science job boards in the 2010s to help bridge the knowledge gap between business and Data teams and enable organizations to be better and more data-informed. Over the last decade, this role has evolved and absorbed more and more facets of data-driven decision-making and has been providing much-needed context and translation for business leadership. This role also plays an important role in interfacing with stakeholder groups such as Marketing, Product, and Strategy to help make all decisions data-centric. With the well-accepted importance of this role and the nimble nature of the set of responsibilities assigned to it, it is essential for all data practitioners to build the “data translation” muscle to excel, succeed, and progress in their roles and career paths.
Addressing the Data Knowledge Gap
Decision-making has been a cornerstone of successful business stories across all industries. Peter Drucker, the notable Management Theory and Practice expert famously said “Every decision is risky: it is a commitment of present resources to an uncertain and unknown future.” In most Modern organizations, data centricity and data-informed decision-making have been agreed upon as proven ways to have reduced risk and ambiguity and business decisions to have a higher likelihood of successful outcomes. Data and marketing executives are tasked with making a series of decisions each day that have far-reaching impacts on an organization’s day-to-day operations and long-term priorities. While data resources are abundant in the current landscape, the process of utilizing these resources is still a struggle. According to a recent study released by Oracle titled “The Decision Dilemma” (April 2023), 72% of business leaders have expressed that the enormous volume of data available and the lack of trust and inconsistencies in data sources have stopped them from making decisions and 89% believe that the growing number of data sources has limited the success of their organizations, despite understanding that decisions that are not backed by data can less accurate, less successful and more prone to errors.
Data-driven decision-making is certainly not a new concept, in fact, the first set of decision models based on data and statistical principles was proposed in 1953 by Irwin D.J Bross distinguishing between the real and symbolic valid and illustrating the importance of measurements and validation. And organizations have consistently evolved over the past few decades to make data investments and have crafted strategies to make data, the center of their risk mitigation and decision-making efforts. Despite having these resources, organizations currently struggle with the unique problem of balancing the appetite for high-quality actionable insights and the availability of resources. A simple “Seesaw” analogy can be used to describe these business circumstances. An excessive appetite for knowledge and actionable insights combined with inadequate data resources may result in Data leaders relying on past decisions, anecdotal evidence, and gut feelings to make decisions. On the other hand, an abundance of data resources combined with less appetite for knowledge can ultimately result in unnecessary data solutions and too many self-serve dashboards being built with no clear strategy to make these resources useful.
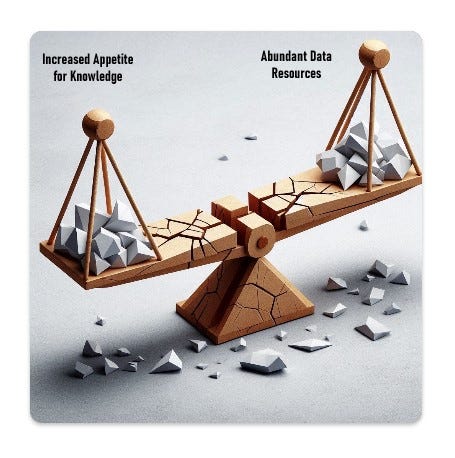
It is becoming increasingly clear that the data knowledge gap is becoming wider despite the availability of abundant data resources. We are increasingly observing a unique situation of a Broken Seesaw, where both Data resources and appetite for knowledge exist, but owing to the lack of efforts to translate the value that the data teams provide to business leaders, both sides of the seesaw get overloaded, eventually leading to broken and inefficient decision making processes in the long run. Valuable data creates impact, everything else sleeps in a dashboard.
Is data literacy the answer?
Yes and No.
Data literacy has quickly become a focal point in the conversation around data insights and delivery, as organizations recognize the importance of equipping employees with the skills to understand and utilize data effectively. The movement gained momentum with research highlighting a significant skills gap among business professionals when it comes to interpreting data. The emphasis on training users to interpret data can sometimes overlook the steep learning curve that is required to build the skill of critically thinking about data evidence and interpreting them in a way that aids in risk mitigation.
Technology barriers are another bottleneck that exists between the data teams and business stakeholders. We can break this bottleneck down into two parts. Firstly, an insufficient analytics tool stack for data analysis can hinder effective data utilization and communication by non-super users of data. Secondly, the lack of training on their use can often lead to misinterpretation and misalignment with other data sources hence hindering the chance to establish a single source of truth. This eventually affects the credibility of the data teams.
A significant drawback of the current emphasis on data literacy is the tendency to place undue blame on users for the shortcomings of data initiatives. When data products fail to deliver value or are met with resistance, the reflexive response is often to assume a lack of user skill or understanding. This perspective overlooks the critical role that business literacy and business context play in effectively communicating the data insights whether it is proving or disproving business hypotheses. Data literacy is a two-way street. Oftentimes, it is the responsibility of the data team members to view the task from the business perspective and understand why they would care about what the data team has to say. Acknowledging and addressing these shortcomings and aligning data initiatives with business goals can lead to more effective and harmonious data-driven cultures.
The Advent of Data Translators — Who were they back then, and who are they now?
One solution that the data industry has adopted to address this data and knowledge gap and the shortcomings of data literacy efforts is the introduction of “Data Translator” roles within organizations. The role of a data translator has evolved significantly over the years, reflecting changes in how organizations utilize data analytics. Initially emerging as a bridge between data scientists and business units, the role was designed to ensure that complex data insights were translated into actionable business strategies.
In the early stages, data translators were primarily seen as intermediaries who could communicate technical findings to non-technical stakeholders, helping to prioritize business problems and ensuring that analytics solutions were aligned with business goals. As the demand for data-driven decision-making grew, so did the importance of this role. By 2019, the role had become more prevalent, with about a third of companies having positions fitting the data translator description. The responsibilities expanded to include not only communication but also ensuring that analytics tools were adopted across enterprises and that data democratization was achieved. Recently, there has been a shift towards integrating these roles into broader functions such as Data Product Owners, reflecting an evolution towards more holistic roles that encompass both technical and strategic responsibilities. This evolution highlights the ongoing need for roles that can effectively link data insights with business outcomes.
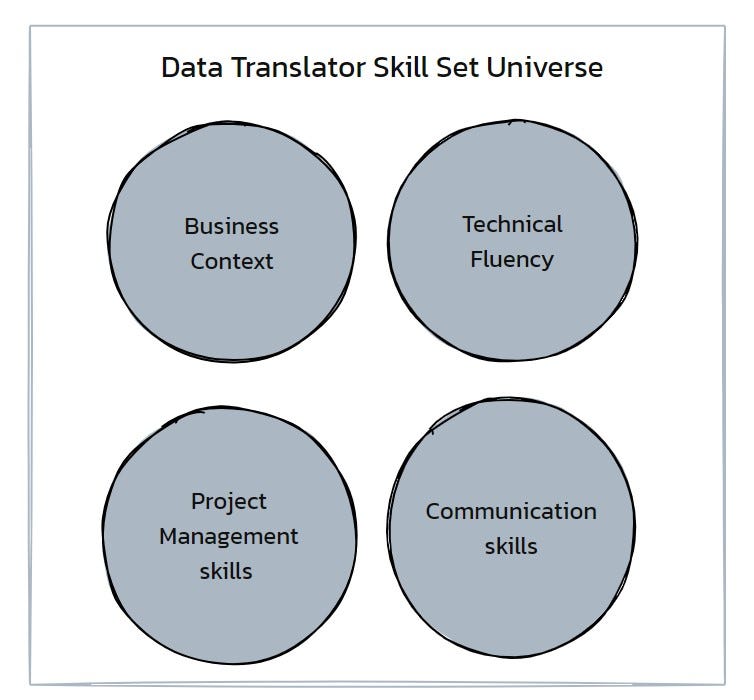
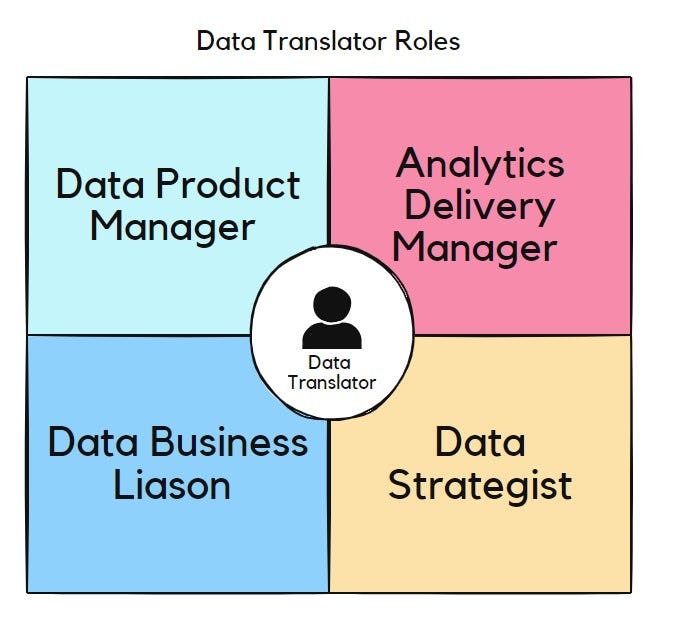
The Data Translator role can take on a multitude of responsibilities depending upon the nature of the organizations they serve. For example, consulting organizations typically assign a dedicated Data Translator who is responsible for translating the provided data solutions to the business audience. Professionals who are hired in-house typically take the form of either dedicated Data Translator resources, Data Product Managers, or Analytics Delivery Managers with the responsibility of ensuring that the Data team’s efforts are utilized appropriately for critical business decisions. Despite having various job titles, Data Translators are tasked with the critical responsibility of proving the value and impact driven by data teams. They accomplish this by focusing on the following key areas:
1. Cost Containment:
Data Translators work as liaisons between the business leaders and data teams by consistently quantifying the impact of the projects delivered by the data team and weighing on the thoughtful allocation of data resources. For example, they may do this by keeping a record of monetary impact and decisions driven by the data teams they support. This record is often helpful in estimating resources for new strategic initiatives and serves as a reference for data solutions that can be replicated for similar problems in new contexts.
2. Strategy and Prioritization:
Data translators have a solid grasp of business goals and priorities and work on aligning their team’s efforts with the broader business objectives. This process often involves identifying projects that not only leverage the team’s skills but also have the potential to influence strategic outcomes. A popular approach to prioritization is using a framework that assesses the potential impact and feasibility of projects. By streamlining the data team’s intake systems and focusing on initiatives that promise significant returns or solve critical business problems, data teams can maximize their usefulness and productivity. In an article explaining the traits of data product managers and translators, Harvard Business Review identified business context, broad technical fluency, project management skills, an entrepreneurial spirit, and the ability to explain data needs and strategy to the rest of the organization.
3. Bridging the Data Literacy Gap
Data Translators work with Governance teams across the organization to establish common data language, definitions, and standards to ensure that all teams are aligned in their understanding and interpretation of data. This ensures that all data efforts are working together cohesively to establish a single source of truth.
4. Stakeholder Engagement
Identifying and prioritizing key stakeholders is essential for data teams to ensure their efforts are aligned with the organization’s strategic goals. Data Translators often accomplish this by using a project management technique called the “Interest — Influence Matrix”. This process begins by mapping stakeholders across two dimensions: their level of interest in data initiatives and their influence on decision-making. High-interest and high-influence stakeholders are considered key players and should be prioritized for regular communication and collaboration. Building strong relationships with these individuals is crucial, as they can champion data projects, help secure resources, and remove roadblocks. For less influential stakeholders, maintaining periodic contact ensures they remain informed without overextending team resources. This type of thoughtful engagement enables data teams to focus their efforts where they can have the most significant impact, driving value for the organization as a whole.
5. Internal Promotion and Outreach
In an increasingly data-centric landscape, the role of Data teams has become significant, yet they are often misunderstood. Data Translators often create roadshows, presentations, and educational materials to share out the Data Team’s achievements and value provided in order to build and maintain credibility and trust across the organization.
Building the Data Translator Muscle
Observing the history and evolution of the Data Translator role has established that, along with data fluency, it is essential to have domain knowledge, Business context, and a solid understanding of organizational nuances such as goals, expected outcomes, and effective stakeholder partnerships to be successful in this role. The nimble nature of this role cannot go unnoticed. Over the past few decades, professionals across the data ecosystem with various job titles have been absorbed into the “Data Translator” roles and responsibilities in different ways. In order to future proof their data careers and be consistently successful and valuable to their organizations, data professionals must build the “Data Translator” muscle.
Practical tips Analysts can follow to empower themselves to be Data translators
Elaborated below, is a non-exhaustive list of practical tips that will help analysts become well-versed in Data Translation.
Curse of Knowledge
The curse of knowledge is a cognitive bias that occurs when a person who has specialized knowledge assumes that others share that same knowledge. This bias makes it difficult for knowledgeable individuals to imagine what it’s like to lack their expertise. Assuming everyone shares the same understanding and background knowledge leads to misunderstandings, wrong assumptions and ineffective communication. This is particularly true when interfacing with teams such as Marketing and Product, where the stakeholders are not necessarily data fluent, but data plays a major role in their projects and campaigns being efficient and fruitful. A data translator must have the unique capability to dissect the problem statement and map it into data points available, make the connections, find answers, and explain it to stakeholders in plain English. Here is a Marketing Analytics example:
Statement 1 (Analyst): Looking at the channel attribution charts, it looks like most of your campaign’s ROAS is negative, but it looks like there is less churn and more engagement, it’s not all wasted effort.
Statement 2 (Data translator): After assessing the marketing dollar spend and returns, it looks like your campaign is losing money in the short term. But looking at the big picture, the users acquired by your marketing campaigns are engaging and returning more hence creating long-term value.
The data translated version of the statement clearly explains the findings and illustrates the long-term impact of the campaign without the Data Analytics jargon.
Move on from business questions to business goals.
Oftentimes, analysts confine themselves to the bounds of their job responsibilities and purely focus on answering business questions. Sometimes, this phenomenon is also an unexpected side effect of organization-wide Data Literacy efforts. Answering business questions limits the insights to a specific problem while focusing on the overall business outcome gives a chance for both the Data and Business teams to look at data insights at a more holistic level. Data Literacy goes hand in hand with Business literacy. Data Translators are always expected to have a working knowledge of the business outcomes so they can tie insights to the overarching goals.
For example,
Business Question: How is my newly launched brand campaign doing?
Answer (Analyst): We had 6000 impressions in 3 days which is 50% higher compared to the last time we ran a similar campaign same time last year.
Answer (Data Translator): The expected outcome of this campaign is to improve brand awareness. We had 3000 net new users visit our website from this campaign. We also measured brand perception metrics before vs. after using a survey poll for these specific users and their opinions and awareness about the brand’s product offerings have improved.
Learn to Zoom out
Learning to zoom out and look at the big picture, and being able to map out individual tasks into overall priorities help Data translators focus their efforts on impactful initiatives. This skill also enables them to learn to build scalable analytics solutions that can be repurposed, eventually leading to time savings and better speed to insight.
Become a good data storyteller
“I didn’t have time to write a short letter, so I wrote a long one instead.”
― Mark Twain
Data storytelling is equal parts science and art. And it is an essential tool in the Data Translator toolkit. It requires a thorough understanding of the problem and solution, constructing a clear, concise, and relatable narrative, and ending with recommendations and insights that can be acted upon. Every data story needs a governing idea that loosely follows an arc. One effective way to arrange the analysis story deck is in the order of Problem, Problem’s Impact, Findings, Recommendations, and Next steps. This ensures that your data story is easy to follow and speaks for itself even when you’re not around to narrate and walk through the whole deck.
The order may look different in repeating tasks such as routine performance updates or retrospective summaries. But for a typical request requiring data insights to aid decision-making, this order is a great starting point. The main thing to ensure in this step is to have accurate and relevant data points that clearly support your story. Apart from that, I have a few tips to help wrap up your Analysis solution neatly. These little details go a long way in presentation delivery and helping the audience remember the key insights.
· Clearly indicate if the key data point being shared is a good sign or a bad sign by using arrows and colors. (Example: A low bounce rate is a good sign, but a low conversion rate is a bad sign.)
· Always add context for any number (data point) shared in the slide by including benchmarking details or trend analyses. (Example: Conversion rate for this month was 12%, this is in line with other SKUs in the same product line and higher compared to the average conversion rate for the same months in the past three years.)
· Tie back the insights to some part of the original business question, goal, and outcome in each slide.
· Including details such as sample size, analysis time frames and important annotations in the footnote will help build trust and credibility.
In essence, a data story can be deemed effective when it leaves the audience informed and inspired to act.
Conclusion
Data Translators perform the critical role of bridging the gap between Data and Business teams. Their skill set is instrumental in proving the worth and impact of data investments, promoting data literacy, prioritizing high-impact initiatives, and protecting Analysts’ time from working on low-value tasks. Organizations and data teams can reap symbiotic benefits by encouraging, incorporating, and nurturing team members with data translator skills.
About the Author :
Nithhyaa Ramamoorthy is a Data Subject matter Expert with over 12 years’ worth of experience in Analytics and Big Data, specifically in the intersection of Healthcare and Consumer behavior. She holds a Master’s Degree in Information Sciences and more recently a CSPO along with several other professional certifications. She is passionate about leveraging her analytics skills to drive business decisions that create inclusive and equitable digital products rooted in empathy.
Bridging the Data Literacy Gap was originally published in Towards Data Science on Medium, where people are continuing the conversation by highlighting and responding to this story.
The Advent, Evolution, and Current state of “Data Translators”IntroductionWith Data being constantly glorified as the most valuable asset organizations can own, leaders and decision-makers are always looking for effective ways to put their data insights to use. Every time customers interact with digital products, millions of data points are generated and the opportunity loss of not harnessing these data points to make better products, optimize revenue generation, and improve customer footprint is simply too high to ignore. The role of “Data Translators” began to emerge in analytics and data science job boards in the 2010s to help bridge the knowledge gap between business and Data teams and enable organizations to be better and more data-informed. Over the last decade, this role has evolved and absorbed more and more facets of data-driven decision-making and has been providing much-needed context and translation for business leadership. This role also plays an important role in interfacing with stakeholder groups such as Marketing, Product, and Strategy to help make all decisions data-centric. With the well-accepted importance of this role and the nimble nature of the set of responsibilities assigned to it, it is essential for all data practitioners to build the “data translation” muscle to excel, succeed, and progress in their roles and career paths.Addressing the Data Knowledge GapDecision-making has been a cornerstone of successful business stories across all industries. Peter Drucker, the notable Management Theory and Practice expert famously said “Every decision is risky: it is a commitment of present resources to an uncertain and unknown future.” In most Modern organizations, data centricity and data-informed decision-making have been agreed upon as proven ways to have reduced risk and ambiguity and business decisions to have a higher likelihood of successful outcomes. Data and marketing executives are tasked with making a series of decisions each day that have far-reaching impacts on an organization’s day-to-day operations and long-term priorities. While data resources are abundant in the current landscape, the process of utilizing these resources is still a struggle. According to a recent study released by Oracle titled “The Decision Dilemma” (April 2023), 72% of business leaders have expressed that the enormous volume of data available and the lack of trust and inconsistencies in data sources have stopped them from making decisions and 89% believe that the growing number of data sources has limited the success of their organizations, despite understanding that decisions that are not backed by data can less accurate, less successful and more prone to errors.Data-driven decision-making is certainly not a new concept, in fact, the first set of decision models based on data and statistical principles was proposed in 1953 by Irwin D.J Bross distinguishing between the real and symbolic valid and illustrating the importance of measurements and validation. And organizations have consistently evolved over the past few decades to make data investments and have crafted strategies to make data, the center of their risk mitigation and decision-making efforts. Despite having these resources, organizations currently struggle with the unique problem of balancing the appetite for high-quality actionable insights and the availability of resources. A simple “Seesaw” analogy can be used to describe these business circumstances. An excessive appetite for knowledge and actionable insights combined with inadequate data resources may result in Data leaders relying on past decisions, anecdotal evidence, and gut feelings to make decisions. On the other hand, an abundance of data resources combined with less appetite for knowledge can ultimately result in unnecessary data solutions and too many self-serve dashboards being built with no clear strategy to make these resources useful.Source: Image illustrated by the author using AI prompts.It is becoming increasingly clear that the data knowledge gap is becoming wider despite the availability of abundant data resources. We are increasingly observing a unique situation of a Broken Seesaw, where both Data resources and appetite for knowledge exist, but owing to the lack of efforts to translate the value that the data teams provide to business leaders, both sides of the seesaw get overloaded, eventually leading to broken and inefficient decision making processes in the long run. Valuable data creates impact, everything else sleeps in a dashboard.Is data literacy the answer?Yes and No.Data literacy has quickly become a focal point in the conversation around data insights and delivery, as organizations recognize the importance of equipping employees with the skills to understand and utilize data effectively. The movement gained momentum with research highlighting a significant skills gap among business professionals when it comes to interpreting data. The emphasis on training users to interpret data can sometimes overlook the steep learning curve that is required to build the skill of critically thinking about data evidence and interpreting them in a way that aids in risk mitigation.Technology barriers are another bottleneck that exists between the data teams and business stakeholders. We can break this bottleneck down into two parts. Firstly, an insufficient analytics tool stack for data analysis can hinder effective data utilization and communication by non-super users of data. Secondly, the lack of training on their use can often lead to misinterpretation and misalignment with other data sources hence hindering the chance to establish a single source of truth. This eventually affects the credibility of the data teams.A significant drawback of the current emphasis on data literacy is the tendency to place undue blame on users for the shortcomings of data initiatives. When data products fail to deliver value or are met with resistance, the reflexive response is often to assume a lack of user skill or understanding. This perspective overlooks the critical role that business literacy and business context play in effectively communicating the data insights whether it is proving or disproving business hypotheses. Data literacy is a two-way street. Oftentimes, it is the responsibility of the data team members to view the task from the business perspective and understand why they would care about what the data team has to say. Acknowledging and addressing these shortcomings and aligning data initiatives with business goals can lead to more effective and harmonious data-driven cultures.The Advent of Data Translators — Who were they back then, and who are they now?One solution that the data industry has adopted to address this data and knowledge gap and the shortcomings of data literacy efforts is the introduction of “Data Translator” roles within organizations. The role of a data translator has evolved significantly over the years, reflecting changes in how organizations utilize data analytics. Initially emerging as a bridge between data scientists and business units, the role was designed to ensure that complex data insights were translated into actionable business strategies.In the early stages, data translators were primarily seen as intermediaries who could communicate technical findings to non-technical stakeholders, helping to prioritize business problems and ensuring that analytics solutions were aligned with business goals. As the demand for data-driven decision-making grew, so did the importance of this role. By 2019, the role had become more prevalent, with about a third of companies having positions fitting the data translator description. The responsibilities expanded to include not only communication but also ensuring that analytics tools were adopted across enterprises and that data democratization was achieved. Recently, there has been a shift towards integrating these roles into broader functions such as Data Product Owners, reflecting an evolution towards more holistic roles that encompass both technical and strategic responsibilities. This evolution highlights the ongoing need for roles that can effectively link data insights with business outcomes.Figure 1 : Dominant themes in Data Translator Skill set universe. Source :Image illustrated by author.Figure 2: The various roles that the Data Translator skills fuel. Source: Image illustrated by author.The Data Translator role can take on a multitude of responsibilities depending upon the nature of the organizations they serve. For example, consulting organizations typically assign a dedicated Data Translator who is responsible for translating the provided data solutions to the business audience. Professionals who are hired in-house typically take the form of either dedicated Data Translator resources, Data Product Managers, or Analytics Delivery Managers with the responsibility of ensuring that the Data team’s efforts are utilized appropriately for critical business decisions. Despite having various job titles, Data Translators are tasked with the critical responsibility of proving the value and impact driven by data teams. They accomplish this by focusing on the following key areas:1. Cost Containment:Data Translators work as liaisons between the business leaders and data teams by consistently quantifying the impact of the projects delivered by the data team and weighing on the thoughtful allocation of data resources. For example, they may do this by keeping a record of monetary impact and decisions driven by the data teams they support. This record is often helpful in estimating resources for new strategic initiatives and serves as a reference for data solutions that can be replicated for similar problems in new contexts.2. Strategy and Prioritization:Data translators have a solid grasp of business goals and priorities and work on aligning their team’s efforts with the broader business objectives. This process often involves identifying projects that not only leverage the team’s skills but also have the potential to influence strategic outcomes. A popular approach to prioritization is using a framework that assesses the potential impact and feasibility of projects. By streamlining the data team’s intake systems and focusing on initiatives that promise significant returns or solve critical business problems, data teams can maximize their usefulness and productivity. In an article explaining the traits of data product managers and translators, Harvard Business Review identified business context, broad technical fluency, project management skills, an entrepreneurial spirit, and the ability to explain data needs and strategy to the rest of the organization.3. Bridging the Data Literacy GapData Translators work with Governance teams across the organization to establish common data language, definitions, and standards to ensure that all teams are aligned in their understanding and interpretation of data. This ensures that all data efforts are working together cohesively to establish a single source of truth.4. Stakeholder EngagementIdentifying and prioritizing key stakeholders is essential for data teams to ensure their efforts are aligned with the organization’s strategic goals. Data Translators often accomplish this by using a project management technique called the “Interest — Influence Matrix”. This process begins by mapping stakeholders across two dimensions: their level of interest in data initiatives and their influence on decision-making. High-interest and high-influence stakeholders are considered key players and should be prioritized for regular communication and collaboration. Building strong relationships with these individuals is crucial, as they can champion data projects, help secure resources, and remove roadblocks. For less influential stakeholders, maintaining periodic contact ensures they remain informed without overextending team resources. This type of thoughtful engagement enables data teams to focus their efforts where they can have the most significant impact, driving value for the organization as a whole.5. Internal Promotion and OutreachIn an increasingly data-centric landscape, the role of Data teams has become significant, yet they are often misunderstood. Data Translators often create roadshows, presentations, and educational materials to share out the Data Team’s achievements and value provided in order to build and maintain credibility and trust across the organization.Building the Data Translator MuscleObserving the history and evolution of the Data Translator role has established that, along with data fluency, it is essential to have domain knowledge, Business context, and a solid understanding of organizational nuances such as goals, expected outcomes, and effective stakeholder partnerships to be successful in this role. The nimble nature of this role cannot go unnoticed. Over the past few decades, professionals across the data ecosystem with various job titles have been absorbed into the “Data Translator” roles and responsibilities in different ways. In order to future proof their data careers and be consistently successful and valuable to their organizations, data professionals must build the “Data Translator” muscle.Practical tips Analysts can follow to empower themselves to be Data translatorsElaborated below, is a non-exhaustive list of practical tips that will help analysts become well-versed in Data Translation.Curse of KnowledgeThe curse of knowledge is a cognitive bias that occurs when a person who has specialized knowledge assumes that others share that same knowledge. This bias makes it difficult for knowledgeable individuals to imagine what it’s like to lack their expertise. Assuming everyone shares the same understanding and background knowledge leads to misunderstandings, wrong assumptions and ineffective communication. This is particularly true when interfacing with teams such as Marketing and Product, where the stakeholders are not necessarily data fluent, but data plays a major role in their projects and campaigns being efficient and fruitful. A data translator must have the unique capability to dissect the problem statement and map it into data points available, make the connections, find answers, and explain it to stakeholders in plain English. Here is a Marketing Analytics example:Statement 1 (Analyst): Looking at the channel attribution charts, it looks like most of your campaign’s ROAS is negative, but it looks like there is less churn and more engagement, it’s not all wasted effort.Statement 2 (Data translator): After assessing the marketing dollar spend and returns, it looks like your campaign is losing money in the short term. But looking at the big picture, the users acquired by your marketing campaigns are engaging and returning more hence creating long-term value.The data translated version of the statement clearly explains the findings and illustrates the long-term impact of the campaign without the Data Analytics jargon.Move on from business questions to business goals.Oftentimes, analysts confine themselves to the bounds of their job responsibilities and purely focus on answering business questions. Sometimes, this phenomenon is also an unexpected side effect of organization-wide Data Literacy efforts. Answering business questions limits the insights to a specific problem while focusing on the overall business outcome gives a chance for both the Data and Business teams to look at data insights at a more holistic level. Data Literacy goes hand in hand with Business literacy. Data Translators are always expected to have a working knowledge of the business outcomes so they can tie insights to the overarching goals.For example,Business Question: How is my newly launched brand campaign doing?Answer (Analyst): We had 6000 impressions in 3 days which is 50% higher compared to the last time we ran a similar campaign same time last year.Answer (Data Translator): The expected outcome of this campaign is to improve brand awareness. We had 3000 net new users visit our website from this campaign. We also measured brand perception metrics before vs. after using a survey poll for these specific users and their opinions and awareness about the brand’s product offerings have improved.Learn to Zoom outLearning to zoom out and look at the big picture, and being able to map out individual tasks into overall priorities help Data translators focus their efforts on impactful initiatives. This skill also enables them to learn to build scalable analytics solutions that can be repurposed, eventually leading to time savings and better speed to insight.Become a good data storyteller“I didn’t have time to write a short letter, so I wrote a long one instead.”― Mark TwainData storytelling is equal parts science and art. And it is an essential tool in the Data Translator toolkit. It requires a thorough understanding of the problem and solution, constructing a clear, concise, and relatable narrative, and ending with recommendations and insights that can be acted upon. Every data story needs a governing idea that loosely follows an arc. One effective way to arrange the analysis story deck is in the order of Problem, Problem’s Impact, Findings, Recommendations, and Next steps. This ensures that your data story is easy to follow and speaks for itself even when you’re not around to narrate and walk through the whole deck.The order may look different in repeating tasks such as routine performance updates or retrospective summaries. But for a typical request requiring data insights to aid decision-making, this order is a great starting point. The main thing to ensure in this step is to have accurate and relevant data points that clearly support your story. Apart from that, I have a few tips to help wrap up your Analysis solution neatly. These little details go a long way in presentation delivery and helping the audience remember the key insights.· Clearly indicate if the key data point being shared is a good sign or a bad sign by using arrows and colors. (Example: A low bounce rate is a good sign, but a low conversion rate is a bad sign.)· Always add context for any number (data point) shared in the slide by including benchmarking details or trend analyses. (Example: Conversion rate for this month was 12%, this is in line with other SKUs in the same product line and higher compared to the average conversion rate for the same months in the past three years.)· Tie back the insights to some part of the original business question, goal, and outcome in each slide.· Including details such as sample size, analysis time frames and important annotations in the footnote will help build trust and credibility.In essence, a data story can be deemed effective when it leaves the audience informed and inspired to act.ConclusionData Translators perform the critical role of bridging the gap between Data and Business teams. Their skill set is instrumental in proving the worth and impact of data investments, promoting data literacy, prioritizing high-impact initiatives, and protecting Analysts’ time from working on low-value tasks. Organizations and data teams can reap symbiotic benefits by encouraging, incorporating, and nurturing team members with data translator skills.About the Author :Nithhyaa Ramamoorthy is a Data Subject matter Expert with over 12 years’ worth of experience in Analytics and Big Data, specifically in the intersection of Healthcare and Consumer behavior. She holds a Master’s Degree in Information Sciences and more recently a CSPO along with several other professional certifications. She is passionate about leveraging her analytics skills to drive business decisions that create inclusive and equitable digital products rooted in empathy.Bridging the Data Literacy Gap was originally published in Towards Data Science on Medium, where people are continuing the conversation by highlighting and responding to this story. deep-dives, big-data-career, data-literacy, data-analysis, data-translation Towards Data Science – MediumRead More


0 Comments